Most brands don’t have the resources to offer 24/7 online customer service and sales support on Facebook Messenger, let alone on their website. Fortunately, chatbots don’t need to sleep (or eat lunch). Facebook Messenger bots can answer customer questions, track packages, make product recommendations, and even close a sale any time of the day or night.
Facebook is the most-used social media platform in the world. If you already have a shop set up on Facebook, you’ve taken the right step to join an ever-growing online marketplace. You’d be missing out on solid sales opportunities if you don’t consider adding a Facebook Messenger chatbot to your team.
Find out how to use Facebook Messenger bots (a.k.a. Facebook chatbots) for customer service and social commerce below. By leveraging Facebook Messenger marketing, you can create a streamlined experience for your customers and followers and stand out from your competition.
Bonus: Download a free guide that teaches you how to turn Facebook traffic into sales in four simple steps using Hootsuite.
What is a Facebook Messenger bot (a.k.a Facebook chatbot)?
A chatbot is a piece of automated messaging software that uses artificial intelligence to converse with people.
Facebook Messenger bots live within Facebook Messenger, and can converse with some of the 1.3 billion people who use Facebook Messenger every month.
Chatbots are like virtual assistants. They can be programmed to understand questions, provide answers, and execute tasks. They can provide a customized online shopping experience and even make sales.
The benefits of using Facebook Messenger bots for business
Meet customers where they are
First, let’s look at some quick stats to set the stage for how much of your potential audience is accessible through Facebook Messenger:
- Chat and messaging are the most-used types of websites and apps, followed by social networks.
- The number of messages sent to businesses on Facebook has doubled in the past year.
- More than 375,000 people from over 200 countries engage with bots on Messenger every day.
- Facebook Messenger has the third-most active users of any app, beat out only by Facebook and Whatsapp
- More than 100 billion messages are exchanged on Meta apps every day.
- People spend an average of 3 hours every month using Facebook Messenger (and 19.6 hours a month using Facebook itself).
- Meta reports the potential advertising audience for Facebook Messenger is 987.7 million people
- Most people (69% in the U.S.) who message businesses say being able to do so improves their confidence in the brand.
The point is that your audience is already using Facebook Messenger, and they expect to be able to interact with your brand there when visiting your Facebook page. Chatbots can increase your rate of response, making it easier for people to get the information they expect in real-time, on a channel they already use.
As a bonus, Facebook Messenger has sponsored ads, which can be targeted to people who have previously been in touch with your Page. Use these ads in tandem with your chatbot to target high-intent customers.
Save time for your team and your customers
Customers expect 24/7 availability, and they hate waiting on hold. They also ask many of the same questions over and over (and over) again.
If you’re spending a lot of time helping people track deliveries, check your return policy, or book appointments, a little automation will go a long way. Customers will be able to access the information they need, even if you’re unavailable.
They’ll save time with instant answers to their questions, and you’ll save time by letting your Facebook Messenger chatbot answer the easy questions, like in this example from Canadian retailer Simons.
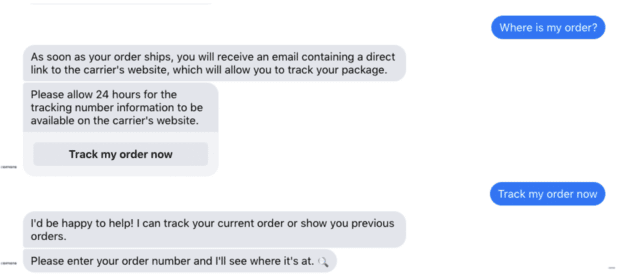
This frees up more time for humans to address more complicated Messenger conversations that go beyond the capabilities of a Facebook chatbot.
Automate sales
Don’t limit your Messenger bots for Facebook to customer service requests.
More than 16% percent of people use social media messaging and live chat services for brand research. And 14.5% say a chat box to speak with a company is a driver of their online purchases. This all leads to real business results: 83% of consumers say they would shop for or buy products in messaging conversations.
With the right script, a Facebook Messenger chatbot can make sales. Conversational commerce allows for personalized recommendations, lead qualification, and upselling.
As your bot greets potential customers, it can identify their needs, ask basic questions, provide inspiration, and direct high-quality leads to your human sales team.
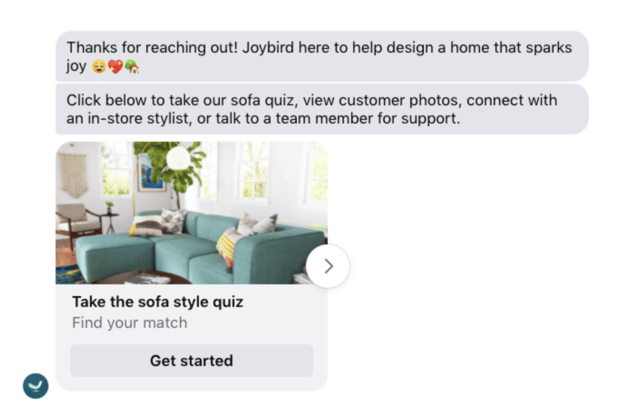
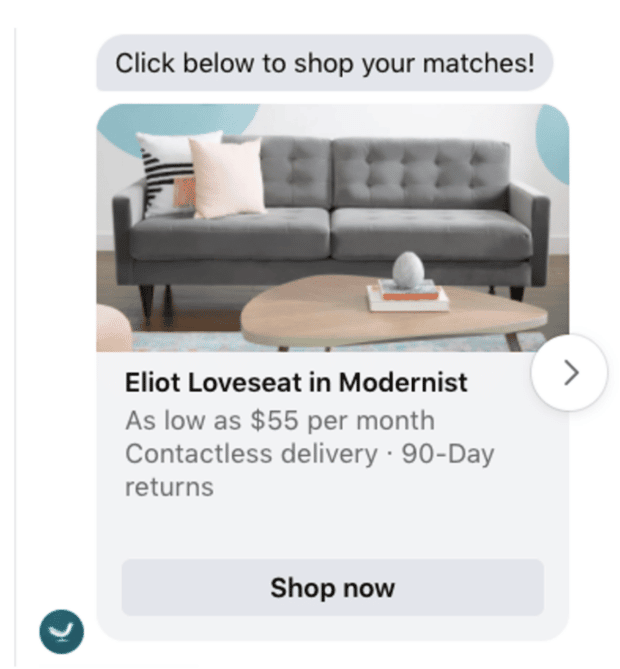
Your Facebook chatbot can also follow up with people who abandon the conversational commerce process, like in this message Joybird’s bot sent 24 hours after completing the sofa-style quiz.
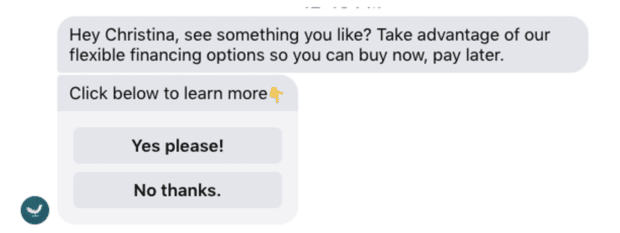
The dos and don’ts of using Facebook Messenger bots
DO set expectations clearly
First, make sure the user knows they’re interacting with a bot. Introducing the bot can be a good way to start. You can even give it a name, like Decathlon does here.
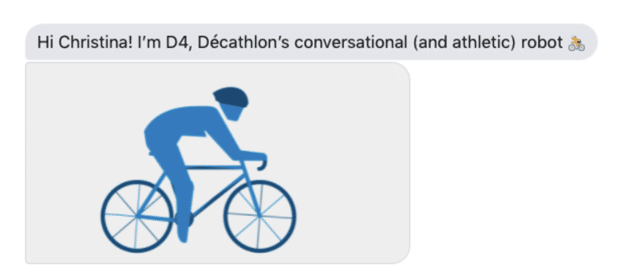
Then, make it clear what the bot can and can’t do. Program your Facebook Messenger chatbot to take the lead in guiding the user through the experience by asking questions or using prompts that move the interaction forward.
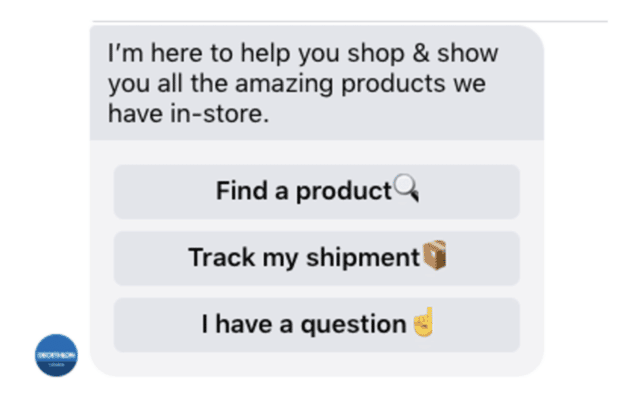
If the bot needs time to process a request, use the typing indicator (three dots) to make sure your customer knows things are still happening, as seen in this example from Tiffany & Co.
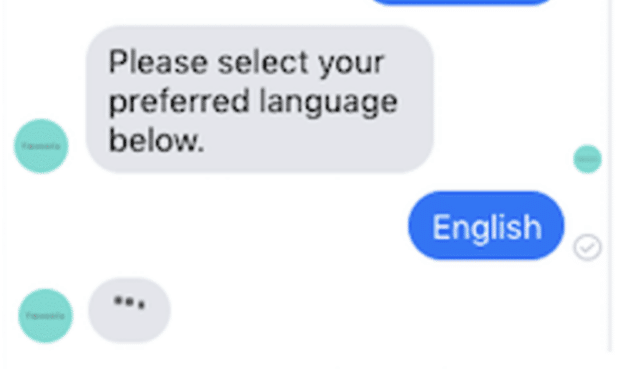
If you need time to reply or pass the conversation along to a person, make that clear, too, and set expectations about when the customer can expect a response, like Bumble’s Facebook bot does here.
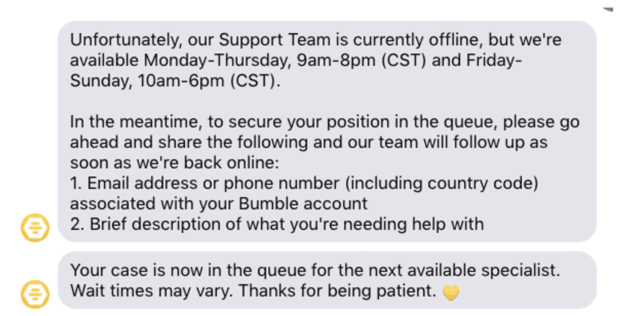
A mini-don’t as part of this tip: Don’t refer to your Facebook chatbot as “live chat” or use other terminology that implies it’s a real person.
DO keep it short
According to Facebook, most people interact with Messenger bots on their mobile devices. Don’t make them read big chunks of text on a small screen or type a lengthy reply with their thumbs.
Buttons, quick replies, and menus can make the conversation flow more easily than asking the customer to type at every stage. Here, KLM provides eight potential options to drive the conversation with the bot.

Allow the customer to type details when needed, but always provide default answers or options to choose from when your Facebook Messenger bot asks a question.
DO maintain your brand voice
While you want to be clear that your Facebook Messenger chatbot is a bot, you want it to sound like your bot. Use turns of phrase your customers expect from your website, and maintain the same general tone. If your brand is casual and friendly, your bot should be too.
That said, keep it simple. Don’t use slang or jargon that will confuse users. Try reading your bot’s prompts out loud to a colleague to make sure they’re clear.
And always use a tone appropriate to the task at hand. If you’re asking someone to provide personal details like a flight number or their address, take a more professional tone.
DO let human agents handle complicated inquiries
A Facebook chatbot’s success depends on its ability to recognize when a human being is needed. Automated conversations are speedy and responsive, but they can’t replace human connection.
Customers should have the option, at any point in the conversation, to connect with a person. Your chatbot should be able to recognize a request for human help, which builds trust, even if it’s outside the expected flow of conversation.
In this example from La Vie En Rose, the bot understands the requests even though it does not flow logically from the bot’s prompt.
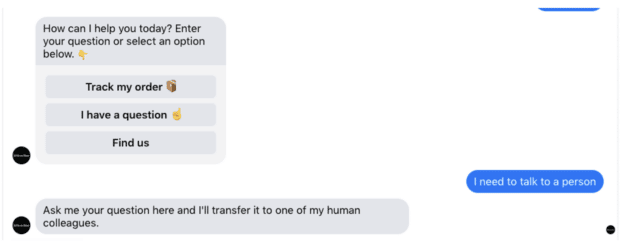
DON’T spam
There’s really only one major don’t when it comes to Messenger bots, and this is it. Don’t spam.
Don’t assume that a customer who reached out for help wants to receive marketing messages. Personalized product recommendations might be helpful, but make sure you have permission before sending them.
Offer people a way to opt-in to ongoing messaging before you contact them. And be sure to offer a clear way to opt out of future communications. Your bot should recognize language that seems like a request to opt out and either ask to confirm or implement the unsubscriber request.

Facebook puts this bluntly in their guidelines for developers: “Don’t change the type of info you send without consent. If people signed up for a specific alert, honor their preferences.”
5 tools for building effective Facebook Messenger bots
1. Streamchat
Streamchat is one of the most basic Facebook chatbot tools out there. It’s meant to be used for simple automations and autoresponders. Rather than managing a whole conversation, it’s useful for out-of-office replies or messages that set expectations about when you’ll be able to respond.
It’s quick to implement and easy to start with if you’re just dipping your toes into the chatbot waters.
2. Chatfuel
Chatfuel has an intuitive visual interface complemented by editable front-end and customization options. While you can build a Facebook Messenger bot for free, a lot of the more complex (and interesting) tools are only available with Chatfuel Pro accounts.
3. Customers.ai
This free tool features a visual chatbot builder for Facebook Messenger designed for non-technical users. You can use it to build Q&A sessions in a Facebook Messenger chatbot.
There’s also a “Chat Blaster” feature, similar to Chatfuel’s “broadcasting” feature, that allows you to send messages to multiple users at once. (Remember: Only do this if you have permission!)
4. Messenger for Developers
If you have the solid coding knowledge required to code your own Facebook chatbot, Facebook provides plenty of resources to get you started. And they are always working with their developer community to come up with new ideas to improve the user experience.
5. Facebook Creator Studio
While it’s not strictly speaking a Facebook Messenger bot, Facebook Creator Studio does allow you to set up some basic automated responses to common requests and events in Messenger. For example, you can set up an away message, provide contact information, or set up a list of FAQs and answers. There’s no artificial intelligence happening here to enable conversation or sales, but you can get some autoresponder functionality to keep Messenger working at a basic level when you’re away from your desk.
Engage with shoppers on their preferred channels, like Facebook, and turn customer conversations into sales with Heyday, Hootsuite’s dedicated conversational AI tools for retailers. Deliver 5-star customer experiences — at scale.