Table of Contents
Welcome to social media marketing in 2025, where trends go from brat to demure in a matter of days.
Brands push creative boundaries while social audiences’ demographics and attention spans tank. Add generative AI, TikTok Shops, and the ever-growing expectation for social commerce to drive business impact to the mix, and it’s enough to make even the savviest marketer’s head spin.
But no need to spiral, friends. We’ve got solutions. Here are the top 15 social media trends you need to know heading into 2025 — and actionable tips to help you bring them to your social media content.
Psst: Since we’re halfway through the year, we’ve refreshed this guide with the latest insights to help you spot which trends are sticking around and which ones are shifting.
Mid-2025 update: Were our trend predictions right?
Now that we’re almost halfway through 2025, it’s the perfect time to do a pulse check on our social trends and see how they’re tracking.
Check out our latest insights to keep your marketing strategy sharp, relevant, and, most importantly, agile:
Threads and X are becoming playgrounds for brand experimentation
We’ve noticed that brands have been using Threads and X as spaces to experiment with tone, humor, and authenticity.
Why? Many organizations just haven’t established clear guidelines for these social media channels (yet), beyond simply aiming to entertain. So this is where they’re ditching polished messaging for unfiltered, real-time social media posts that click with their audiences.
Vibe shifts are replacing fast-moving trends
“Vibe” culture is on the rise, marking a shift from fleeting trends to slower, mood-driven moments. And marketers are evolving their trend-hopping strategies accordingly.
They’re using automation, social listening, and AI to decode the mood and energy behind trends (not just sentiment) to help them curate longer-lasting emotional experiences that strengthen brand identity — not diminish it.
Transparency is the new creative currency
AI is the trend, but the real power move is sharing your prompts. Marketers have embraced AI so much that they’re now creating a culture of teaching and transparency.
They’re no longer pretending they didn’t use AI to get that polished output and instead showing their peers how they got there.
And while we’re on the subject of AI trends, the em dash continues to be a hot topic online — proof that even punctuation can go viral and get people fired up. (We’re team em dash — clearly.)
ChatGPT’s launch in late 2022 may have signaled the start of the AI revolution, but AI adoption only kicked into high gear in 2024. These days, content creators and social media marketers are embracing AI to streamline content creation and inform, inspire, and refine every part of the process.
Social network platforms, in particular, are leading the charge with some impressive AI-driven releases — and we’re pretty sure this is just the beginning.
Meta’s AI, launched in the spring of this year, offers everything from content suggestions for creators to real-time answers to customer questions. It can even generate tailored replies and insights during conversations, making it a powerful tool for both customer service and community engagement.
LinkedIn, meanwhile, creates collaborative articles by pairing AI-generated topic suggestions with contributions from relevant LinkedIn members. The resulting articles benefit both the platform and its users: members get to position themselves as thought leaders, while LinkedIn gets content that keeps their community on the platform longer.
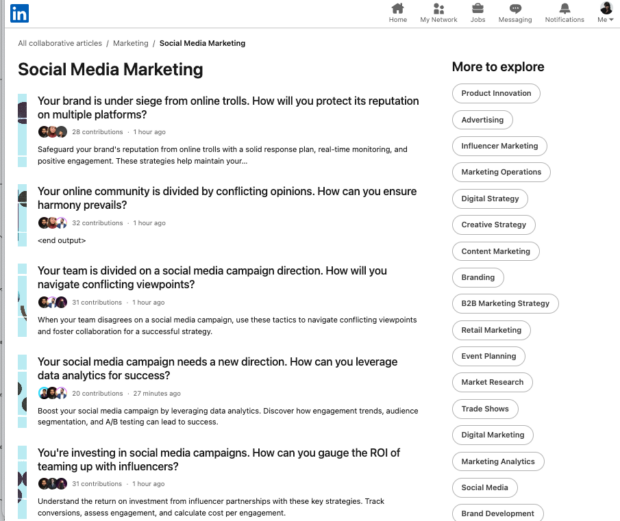
It’s not just Meta and LinkedIn, either. X (Twitter) is using tweets to train its AI, TikTok’s Symphony Assistant helps creators explore trending topics, and Canva’s AI tools can literally create custom designs from a description.
At Hootsuite, we’re seeing firsthand how AI can transform a marketing strategy — and we’re adapting our features in response. Tools like OwlyWriter AI make social media content generation easier than ever, while our social performance score functionality uses AI to provide a clear, weekly snapshot of your content’s performance.
If it feels like everyone is suddenly using AI, that’s because they are.
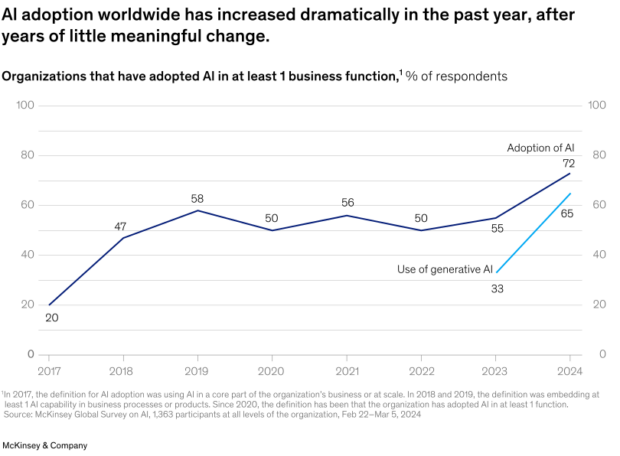
For respondents in Hootsuite’s Social Media Trends Report, it’s no longer a question of if you should use AI but how you’ll use it. And that’s where things get interesting.
While we’re all familiar with using ChatGPT to shortcut the writing process, Hootsuite’s digital marketing Trends Report shows that AI is no longer just a helpful tool for content generation.
Instead, marketers are using AI to refine their marketing strategy, turn messy notes into organized presentations, and brainstorm new ideas. It’s a thought partner, not a job-stealer.
TL;DR? AI isn’t something to be wary of; it’s a critical tool to master if you want to stay competitive.
To-do list:
- Explore AI tools on each platform. If you haven’t already, start playing around with AI tools on top social platforms. Which ones fit your brand’s goals and can genuinely enhance your processes?
- Practice your prompts. AI’s smart, but it still needs your guidance. Spend time experimenting with different ways to phrase prompts for content generation, analysis, or engagement ideas to get the best results.
- Train your team. Don’t keep your AI wisdom to yourself! At Hootsuite, our team regularly shares tips to help improve our use of these tools, and together, we’re all getting a lot better at incorporating AI into our workflows.
2. Social marketing = performance marketing
It’s easy to view social media as “just” a channel for engagement and brand awareness, but its potential as a revenue-driving performance channel is growing.
What does this mean?
Performance marketing is all about driving measurable business outcomes (e.g., sales, leads, and customer acquisition) at the best possible ROI.
Until recently, connecting social media to these business metrics has been challenging. Success on social was typically measured by “vanity metrics” like likes, comments, and shares.
While important, these indicators don’t have a crystal clear connection to revenue. This often left social marketers feeling underappreciated and overlooked in strategic business conversations. It also made securing stakeholder buy-in for new hires, better tools, and higher budgets difficult.
But don’t sweat! The good news is that this is finally changing.
Thanks to tools like social listening and advanced social media analytics, SMMs can now prove the ROI of their work and link social activity to real business impact.
With real-time insights about customer sentiment, emerging trends, and competitors’ weaknesses, you can go from this:
Our social posts drove 2,735 likes and 842 comments this month.
… to this:
Social listening identified customer complaints about our competitors’ lengthy customer service wait times. So we created a ‘No-Wait Guarantee’ campaign, which we launched as paid and organic social posts. It drove 35% more traffic to our site and a 10% lift in new customer sign-ups.
In-depth social data can inform smarter marketing budget allocation — but also improve product development, customer service, and corporate strategy.
In the coming year, more brands will lean on social media as a core performance channel, driving measurable growth and producing invaluable business intelligence. SMMs skilled in listening and data-driven strategy will not only improve their results but also earn a “seat at the table” and a larger share of their organization’s marketing budget.
To-do list:
- Understand the performance loop. Every insight from social should guide an action that drives business results. This, in turn, will generate more insights.
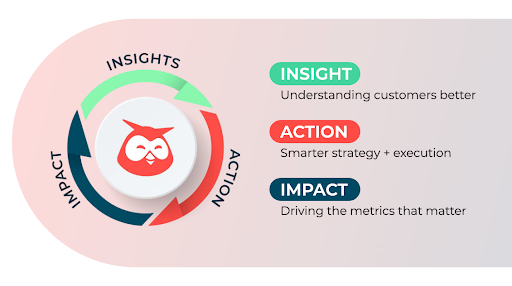
- Prepare your sales team for social leads. As your social strategy starts delivering more qualified leads, make sure your sales team is ready to handle them with the same priority as leads from other channels.
- Integrate social data into your CRM. Tracking social data alongside other customer information in your CRM will provide a fuller picture of your leads, empowering better-targeted sales and marketing efforts.
- Elevate your reporting with tools that connect social media channels and search engines. Go beyond vanity metrics (like likes and follows) to capture the business impact of social. Track how social insights fuel campaigns that drive traffic, conversions, and customer retention. Your tech stack should include a social listening tool, a social media analytics tool, a cross-channel analytics tool (e.g., GA4 or Adobe Analytics), and a business reporting tool (e.g., Tableau).
3. Trendjacking vs. trend detox
Social trends (or, more and more often, micro trends) come and go so fast that marketers rarely have time to make an informed decision about whether to join in or step back.
While 82% of social media managers report being up-to-date with current trends, fewer feel participating is always a good idea. And a healthy dose of apprehension is called for.
Brands that jump from one viral moment to the next without an underlying strategy risk appearing inauthentic — and, ultimately, annoying their audiences (or disappearing in a sea of nearly identical posts).
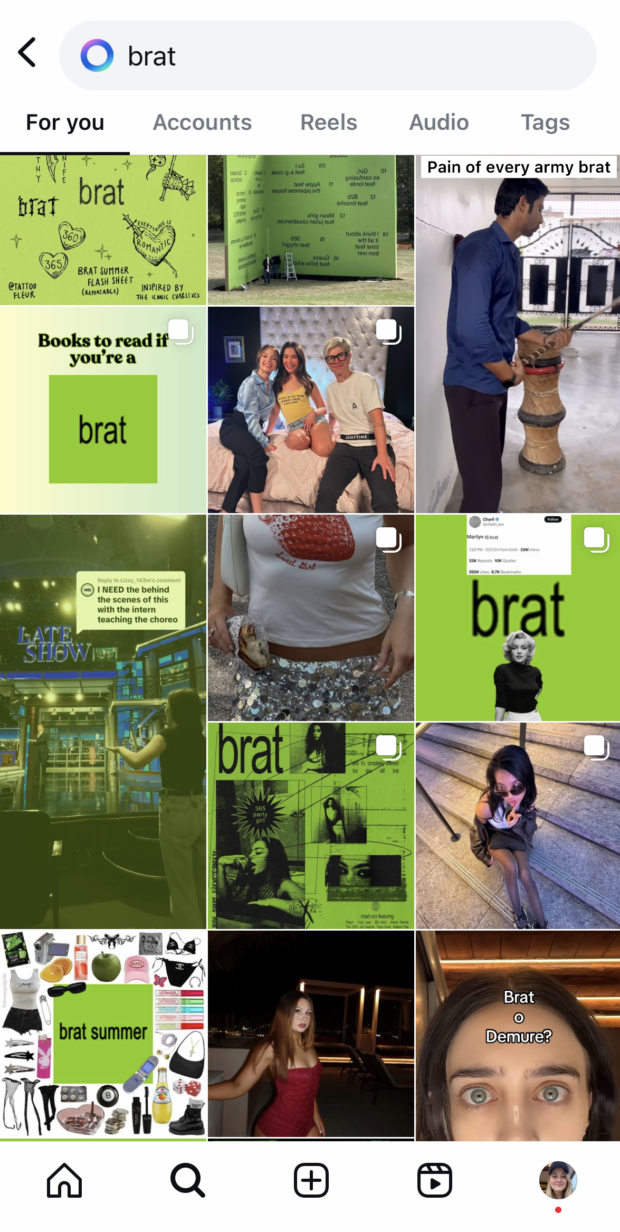
So, how do you know when to join in on a trend?
The two extreme approaches are trendjacking (folding all major trends into your social strategy) and the polar opposite, a trend detox. (The latter is Jack Appleby’s concept of taking deliberate breaks from current events to produce original content true to a brand’s identity and goals.)
Luckily, you don’t have to choose between extremes. Digging into context and making strategic, data-informed decisions is the smart in-between. And social listening tools can help you do just that.
Social listening doesn’t just help brands spot emerging trends. It also helps marketers gauge the relevance and sentiment of a trend, predict its longevity, and identify key creators contributing to the viral moment.
With these insights, brands can make informed decisions about which trends to engage with and when to step back.
In either case, flexibility is key. 27% of social marketers say they regularly adjust their content strategies based on current trends, whether that means joining a viral moment or strategically hitting the brakes.
Currently, only 29% of social marketers use social listening for trend monitoring — but the majority of those who do report seeing a positive impact on their business.
In 2025, expect to see fewer brands diving headfirst into every hot trend. Instead, more will be using social listening insights to carefully pick their moments — and gauge sentiment, track user-generated content, and evaluate whether a trend aligns with their marketing strategy or not.
Whether you’re hopping on the latest YouTube Shorts, Reels, or trending memes, being strategic is essential.
To-do list:
- Set guidelines. Make sure your social media strategy includes a framework for when you should hop on a trend. Plus, if trendjacking is part of your strategy, leave some space in your social media calendar for when a relevant trend pops up.
- Analyze trends thoroughly. When a trend gains traction, use social listening tools to assess its sentiment, lifespan, and potential impact.
- Occasionally, take a break. Stepping back from some trends will allow you the time and focus to create original content centered around your brand’s personality, values, and goals.
4. Brands are building community in the comments
We’ve all seen it — the trending TikTok with a comment section full of blue-checked accounts whose connection to the content seems tenuous at best. Ariana, what are you doing here?
It’s nothing new; brands have been using comments to boost awareness for ages. In fact, there are entire TikTok accounts dedicated to the practice.
But while in the past it seemed like brands would comment on any and every post, in 2025, those outbound engagements will become much more strategic.
Consider Dove, which has been using comment sections to amplify its message of body positivity and self-care. In an interview with Marketing Brew, the social strategists who work on the Dove account explained their approach: it’s all about engaging directly with customers and building positive brand sentiment.
Before commenting, they look at the age of the video, the “volume and velocity” of comments, and the number of brands already commenting. If the TikTok passes the vibe check, Dove comments, allowing the brand to both build community and increase visibility in prime social real estate.
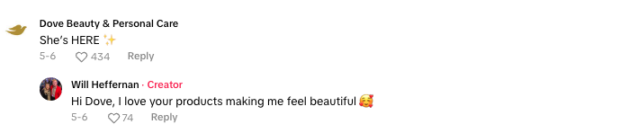
In other words, the comment section is like a mini ad space — if you’re showing up authentically, it can pay off big time.
Hootsuite’s Social Engagement Coordinator, Paige Schmidt, has also noticed that outbound engagements are evolving.
“These days, we’re seeing more strategic thinking: brands are considering which posts to reply to, the tone they’ll use, and which platforms to focus on,” Schmidt explains. This shift isn’t just about visibility — it’s about learning from your audience and shaping brand perception.
If you’re considering joining the conversation (and we’ve always said that social is a conversation, not a one-way broadcast), think of it in terms of relevance, not volume. When done right, thoughtful comments can boost brand recall and build connections without coming off as unplanned or opportunistic.
To-do list:
- Define your outbound engagement strategy. Decide on your tone, which platforms you’ll focus on, and what type of content is most likely to attract your target audience. You might also want to decide on some no-go zones; Dove, for example, doesn’t comment on posts made by underage creators.
- Set clear engagement goals. Are you trying to increase brand awareness? Build relationships with your community? Drive sales? (Actually, we wouldn’t recommend that last one). Make sure you know what you’re trying to achieve before you start commenting.
- Keep an eye out for opportunities. Yes, this means you can officially say scrolling on TikTok is an important part of your job. Just pick your posts carefully and keep your engagements authentic — how can you add value to the discussion?
- Use social listening to track your success. Track metrics like mentions and brand sentiment to see if your outbound strategy is working.
5. Personality matters more than consistency
Traditional marketing made us believe that cross-channel consistency is essential to brand integrity. However, the evolution of social media is proving that audiences respond better to authenticity and relevance than to rigid uniformity in voice and style.
Hootsuite’s 2024 trends survey revealed that above all else, people want to be entertained on social media. Last year, we found that brands were struggling to meet this expectation, focusing too much of their social strategies on products and services. This not only made their content less engaging — it also negatively affected ROI.
Recently, creative marketers have been less risk-averse. And brands that choose to adopt separate content strategies for different social platforms (rather than rigidly enforcing style guidelines across all channels) are seeing impressive results.
This trend aligns with TikTok’s 2024 forecast on “creative bravery,” which encouraged brands to be bold, go big, and embrace platform-specific styles. The inevitable side-effect of this approach is looser brand consistency.
In the last year, 43% of organizations tried out a new tone or personality on social, with some bold enough to diverge significantly from their standard brand voice.
Hever Castle’s playful Instagram posts or the official TikTok account of the Paralympics are great examples of organizations finding unique, platform-appropriate voices — and audiences rewarding these efforts with engagement.
While most organizations aren’t exploring creative extremes (not everyone can pull off Nutter Butter’s abstract humor), even subtle voice adjustments are helping brands blend more seamlessly into each platform’s culture and feel like part of the conversation.
In 2025, expect more brands to push creative boundaries on social and create stronger connections with new and existing audiences. We’re here for it!
To-do list:
- Relax your brand guidelines (but not entirely). Define non-negotiables and identify the brand elements you’re willing to loosen on social. Some strict guardrails can inspire creativity while allowing experimental content to still reflect your values.
- Set new success metrics. Embracing new personas calls for platform-specific KPIs. This may be the perfect moment to lean into “vanity metrics” like engagement. Spikes in likes, comments, and sentiment reveal if audiences are responding to the new voice.
- Get leadership on board. For some decision-makers, the idea of creative shifts without immediate ROI may seem risky. Emphasize that social engagement drives brand loyalty and potential purchases. A follower today can be a customer tomorrow.
6. You need to get on Reddit
Known for its wide range of niche, community-driven subreddits, Reddit is the place to be for authentic, peer-driven engagement.
And guess what? It’s only gaining momentum.
ICYMI: Reddit’s global user base surpassed 1.22 billion in 2024, making it a major player in the social media landscape.
As traditional marketing methods continue to lose their steam, Reddit is becoming one of the most powerful platforms for authentic engagement.
It’s also the platform that users trust the most when learning about new products and brands:
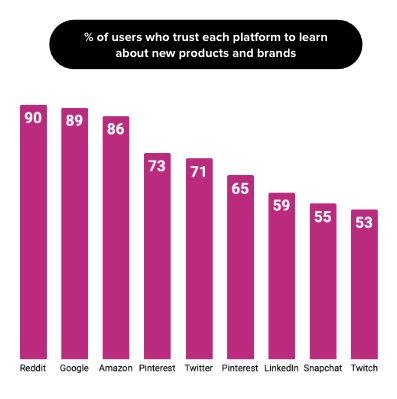
That’s because, unlike platforms that rely heavily on paid ads, Reddit thrives on organic conversations and peer-vetted recommendations. It’s where people go to talk about products, share experiences, and give honest feedback.
For marketers, that means Reddit is a goldmine for insights. You can use social listening to track what users are saying about you and your competitors — or you can jump in and engage with them directly.
But here’s the catch: Reddit is not a place for pushy sales tactics. It’s a space where authenticity and transparency matter. Marketers who succeed on Reddit get there by building relationships through real, helpful conversations rather than simply pushing products.
Take brands like The Washington Post and the NBA. They’ve leveraged Reddit’s communities to connect with users on a deeper level, building trust while addressing pain points and providing value.
Follow their lead to foster real connections with existing Reddit communities and build trust over time.
To-do list:
- Monitor key communities for insights. Start by lurking in subreddits that are relevant to your brand or industry. Pay attention to what people are talking about and take note of their pain points. Use these insights to inform your strategy.
- Engage authentically. Don’t just drop an ad and leave. Reddit is all about real conversations, so focus on providing value rather than promotion. Share helpful tips, offer advice, and build relationships, not transactions.
- Make Reddit a long-term play. Think of Reddit as a marathon, not a sprint. Build your presence slowly by contributing over time. Don’t get discouraged if you don’t see immediate results from a one-off campaign.
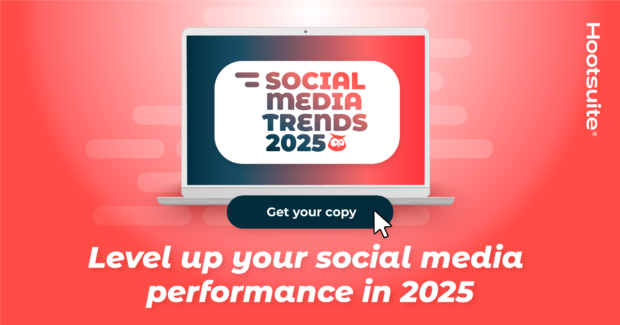
7. TikTok is becoming the real “everything app”
So far, the North American market has yet to replicate the runaway success of “everything apps” like WeChat or KakaoTalk. Still, while some tech giants have tried to position their platforms as the next go-to app, we think TikTok might actually be doing it.
With the launch of TikTok Shop, the app has transformed from a social platform to a full-fledged marketplace. Users can now discover, shop, and buy directly from their favorite creators — and TikTok is set to generate a whopping $17.5 billion in ecommerce sales in 2024, proving it’s a serious player in the online shopping world.
Beyond shopping, TikTok is also the birthplace of nearly every major social trend. The app’s influence on pop culture is so intense that it has essentially created a new kind of “micro trend” cycle.
As soon as one trend peaks, another is already on the rise, which means brands that can tap into these trends early can gain significant visibility — but you have to move quickly. (If you haven’t posted your demure or mindful content yet, maybe ditch that post)
And yes, we know TikTok is facing regulatory challenges that could impact its long-term growth. The platform has already been banned on government devices in Canada and may face similar restrictions in the United States.
While a full consumer ban hasn’t yet been enacted, these moves reflect an increasing focus on privacy concerns around the app, which may shape its future accessibility and usage.
Those concerns aside, we firmly believe that TikTok is redefining what it means to be an all-in-one platform. As the first “everything app” with widespread North American adoption, it offers users a pretty primo combination of social interaction, entertainment, and shopping.
To-do list:
- Experiment with TikTok Shop. If you haven’t already, explore how TikTok Shop might work for your brand. Unfortunately, it’s not available in all markets yet, but if you do have access, it’s time to start using it.
- Stay on top of trends. Don’t miss the next micro trend. Use tools like TikTok’s Creative Center or Hootsuite’s social listening features to catch emerging trends early and align your brand with what’s hot right now.
- Work with UGC creators. If you can’t move fast enough, partner with someone who can. Many creators excel at viral content and can naturally integrate your brand into the next hot trend.
8. Gen Z is the new golden audience
Marketers are putting their money where their future is — and in 2025, that future is Gen Z.
Known for their tech-savvy ways, quick wit, and meme-fueled humor, Gen Zers control over $450 billion in spending power globally. If your brand isn’t paying attention to them, you’re missing out.
But here’s the thing — Gen Z shops differently. Remember, this is the generation who grew up on their phones but rarely uses them to talk. It’s only fitting that, full of contradictions, they wouldn’t follow the same buying rules as Millennials or Boomers.
Enter B2Z™ marketing.
Coined by Hootsuite’s CEO Irina Novoselsky, the term hints at how Gen Z is reshaping the buying journey, even in B2B industries.
Gen Z’s product discovery process starts and ends with social media, especially platforms like Instagram and TikTok, where creators, influencers, and viral trends influence their purchasing decisions.
In fact, 46% of Gen Z begin their B2B search on social, not Google. Think of them as the self-service generation — everything they want needs to be at their fingertips.
Irina says, “They’re a TikTok generation with 6-second attention spans but will research 13 pieces of content before reaching out to a sales rep.”
Gone are the days of traditional ads or relying on Facebook to reach this demo. To connect with Gen Z, brands need to be creative, authentic, and meet them where they are.
“For companies, it’s time to rethink how you show off your products — ditch the demos and build intuitive platforms that let Gen Z buyers find the value themselves, at their own pace,” shares Novoselsky.
Take, for example, how Chili’s taps into viral trends on TikTok, like this Brat summer call-out:
Or this spin on a viral sound with a slew of Chili’s menu suggestions:
Both posts racked up hundreds of thousands of views — plus chatty comment sections flooded with Gen Zs responding to the playful yet relatable approach.
What’s more, these social efforts helped drive business impact: 15% increase in same-store sales for the quarter.
So, what does this mean for your brand? If you want to break through (and sell) to Gen Z, your strategy has to evolve.
With social media driving brand discovery and research, Gen Z expects businesses to meet them where they are. The days of downloading demos or meeting with sales reps to find out more are numbered; Gen Z wants all of this information upfront (and on social) to make their purchasing decisions
To-do list:
- Make your brand fit into Gen Z’s digital-first world. This generation spends most of their time online, so that’s where your brand needs to be too. Whether it’s through interactive content, gamified experiences, or easy-to-digest visuals, make sure you’re catering to their digital lifestyles.
- Ditch ads and embrace community content. Gen Z isn’t interested in traditional ads. Focus on creating organic content that sparks conversation and builds community. Engage with your audience in an authentic, relatable way that feels like a conversation, not a sales pitch.
- Collaborate with influencers they trust. Gen Z is heavily influenced by creators — partner with micro-influencers who have a genuine connection with their followers.
9. Short-form video for long-term wins
Look, we’re not too proud to admit when we’re wrong. Last year, we were pretty sure that long-form video was about to make a big comeback. This year, we can admit that short-form video continues to reign supreme.
Platforms like TikTok, Instagram, YouTube, and LinkedIn have embraced short-form video as the best way to capture attention and increase engagement. With our shortened attention spans and well-honed scrolling habits, we want content that’s quick, engaging, and instantly shareable.
Video isn’t just for B2C brands, either. LinkedIn is seeing huge success with short-form video, noting that video content is now its fastest-growing format. No matter your industry, you can create videos that go beyond entertainment to inform and connect with your audience in seconds.
And while TikToks can now be up to 60 minutes long, consider that an outlier. The average TikTok video may be getting longer, but it’s still under a minute.
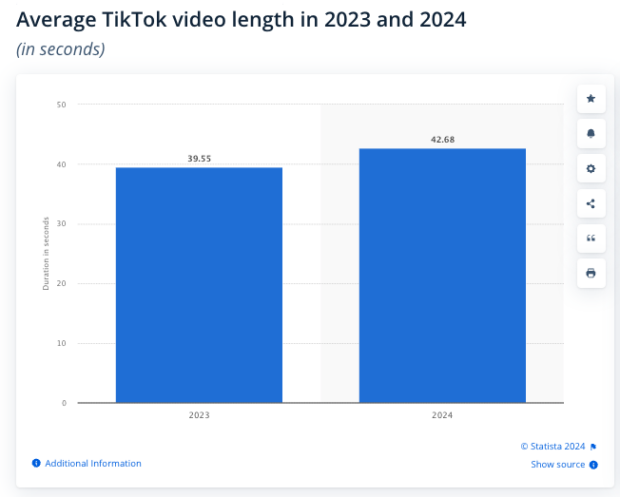
Even Instagram Head Adam Mosseri confirmed that Instagram won’t pivot to long-form video since the format doesn’t align with the platform’s connection-focused primary goals.
Connection really is the name of the game when it comes to short-form, too. Entertainment companies that used to dish out copyright strikes like nobody’s business now upload their own content to video platforms because they know that virality requires access.
For all the talk of hyper-personalized FYPs and super-sophisticated algorithms, the same short-form content tends to bubble up to the top, trending across feeds and reaching users everywhere, regardless of location.
Brands that tap into these major trends can ride a HUGE wave of visibility as users share what resonates with their networks. Our feeds may be personalized, but we’re still drawn to content everyone else is watching. The shared brainrot is truly mind-boggling — unless we’re totally delulu.
What does this mean for brands, though? Consider short-form video an ideal opportunity to build relevance, spark conversations, and capitalize on shared cultural moments.
To-do list:
- Experiment with video lengths. Any video under 90 seconds can be considered short-form, but that leaves a lot of room to play with. Test different lengths to see what resonates best with your audience.
- Your hook is everything. The first few seconds of your video (aka the hook) are critical. You don’t want to lose your audience before you get to the good stuff, so test a variety of audio and visual hooks to see what captures attention fastest.
- Dig into your results. Tools like Hootsuite Analytics can help you pinpoint what’s working and use these insights to iterate quickly.
10. Social media is the new prime-time show
Forget traditional TV — social media is entering its entertainment era, and it’s time for brands to start rolling. Ready, set, action!
Platforms like YouTube, Instagram, and TikTok have become the new “cable” destination, where users can binge-watch TikToks instead of flipping between channels.
In fact, while traditional TV viewership is declining (cable TV consumption dropped from 34.4% in 2022 to just 29.6% in 2023), social media use is on the rise.
The average American is expected to spend nearly 8 hours a day on social media by 2025, up from 7 hours and 19 minutes in 2022.
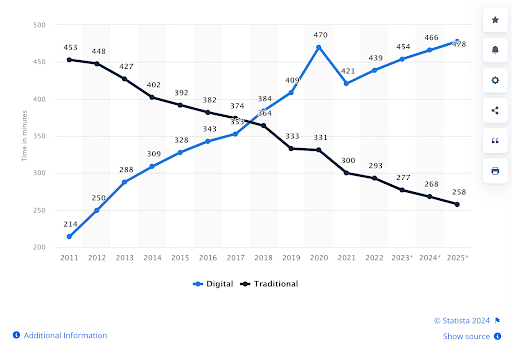
Take Who TF Did I Marry on TikTok, for example — a 50-part series that amassed millions of views per clip.
It wasn’t just a viral hit; it was a masterclass in keeping audiences engaged with episodic storytelling, not dissimilar to your favorite TV show.
And brands took note. Ahead of the 2024 election, Vogue even dedicated a TikTok playlist, Jack Reacts, for their political correspondent Jack Schlossberg to contribute bite-sized political commentary.
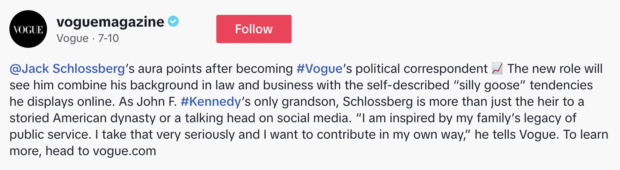
This shift points to our increasingly short attention spans and the surge of platforms heeding the call with Tinsel Town-quality entertainment — offering unique ways to keep users engaged.
So, if your brand has a story to tell, it’s time to adopt a Hollywood mindset.
The social feed is the new appointment television, and it’s time for brands to claim their spot.
To-do list:
- Skip the fancy production — keep it raw and real. You don’t need a Hollywood budget to make waves. Shoot with your phone, focus on a killer hook, and let the story speak for itself.
- Think in episodes, not one-offs. Social media is the new TV, so treat your content like a series. Plan your posts as episodes that build on each other, encouraging your audience to come back for the next “chapter.”
- Use cliffhangers. Just like your fav show, tease your next post and leave your audience wanting more. Whether it’s a product launch or a behind-the-scenes look, create suspense and excitement around what’s coming next.
- Stay consistent. Keep a regular posting schedule, like a show’s airtime. The more you post consistently, the more your audience will expect and look forward to your content.
We’ve been banging the social SEO drum for a few years now, but guys, that’s because it’s really important. Like, really.
As platforms continue to introduce AI-driven features, the way users discover content is only continuing to evolve. This means that now, you’ll also need to get good at AIO, or artificial intelligence optimization.
Just like Google’s AI search, which summarizes information on search results pages, AI-generated social search results deliver content summaries that provide direct answers to user queries.
Take TikTok’s new(ish) search highlights. This feature uses AI to condense search results into quick, easy-to-scan insights that appear above any video content.
“Oh no,” you’re thinking, “Now no one will watch my matcha latte tutorials!” Not quite.
TikTok’s AI search generates a snapshot of what users are looking for, but it also provides links to relevant content. If you want your videos to appear here, you’ll have to ensure that your content is set up to provide the clear, to-the-point answers your audience wants.
Similarly, Meta’s new chat-style AI search uses conversational prompts instead of traditional simple search phrases. So, brands need to think in terms of answering questions with their content, not just dropping keywords into captions.
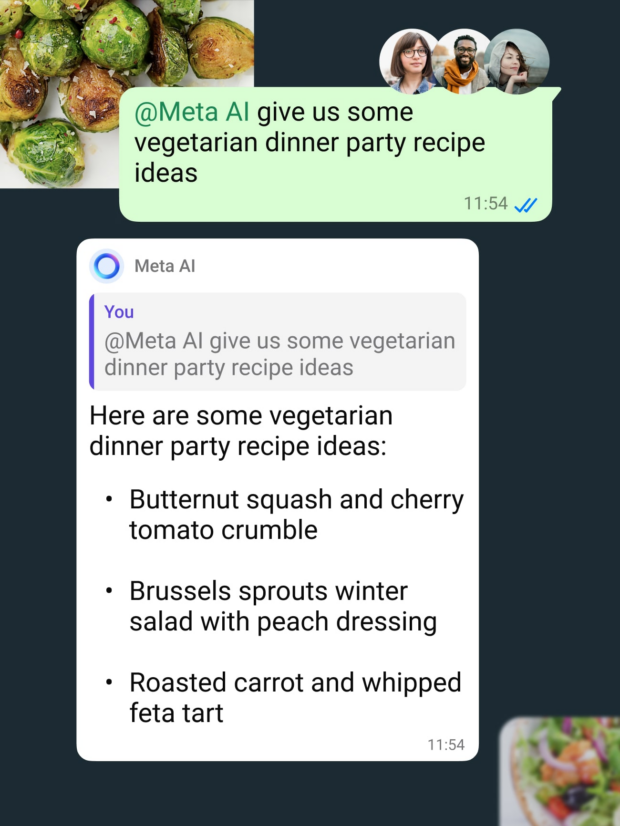
It’s not just about AI search, either; local SEO is also stepping into the social SEO spotlight.
Instagram and TikTok both offer searchable map features that help users find businesses and popular locations nearby, offering relevant brands a huge opportunity.
To optimize for this feature, make sure you’re adding location tags, including all of your business details in your profile, and using area-specific hashtags to boost visibility. That way, when users browse the map feature, those purchase-ready potential customers will find you first.
Adapting to these AI-powered features means making sure your content isn’t just visible but useful and answer-driven. Think of it as a chance to become a go-to resource in your industry, showing up where your audience is asking questions and looking for the best answers.
To-do list:
- Answer user questions. Structure your social posts around common questions people in your industry are asking. Give clear, direct answers that AI can pull into search summaries.
- Make your content easy to scan. Use bullet points, short paragraphs, and lists to make your content easier for AI to grab and summarize.
- Optimize for local search. If you’ve got a physical storefront, use location tags, business categories, and relevant hashtags to appear in map-based searches and local recommendations.
- Keep it conversational. Think about how people naturally ask questions in chat-style prompts, and let that tone guide your content.
SMMs have known that shares are an important engagement metric for some time. But just this summer, Instagram Head Adam Mosseri revealed that they’re also one of the most important ranking signals for the Instagram algorithm.
This means that DM shares per reach now play a more crucial role in building Instagram visibility than traditional metrics like likes or comments. So, if you want to grow your reach — by getting better placement on more followers’ feeds or showing up in the Explore tab — you better start creating sharable posts.
Online retailer SSENSE does this by posting memes featuring their products:
At Hootsuite, we publish original research that marketers share with their teams and peers:
Getting in the algorithm’s good graces is just one benefit of creating sharable content. A recent study by EMARKETER shows that 10% of social shoppers’ purchases are inspired by the content they see in their DMs and group chats.
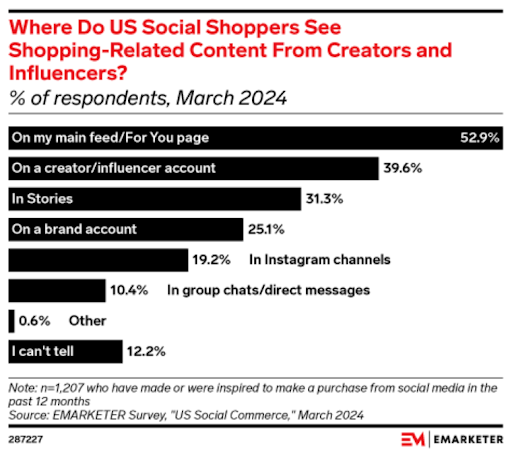
While you wait for your content to spread organically through DMs, you can set up an Instagram broadcast channel — a one-to-many messaging tool that works like a big group chat for your brand’s most engaged followers.
Fashion and beauty brands, including Jaquemus, Fenty, and Cult Gaia, are seeing success using broadcast channels to build exclusive, engaged communities.
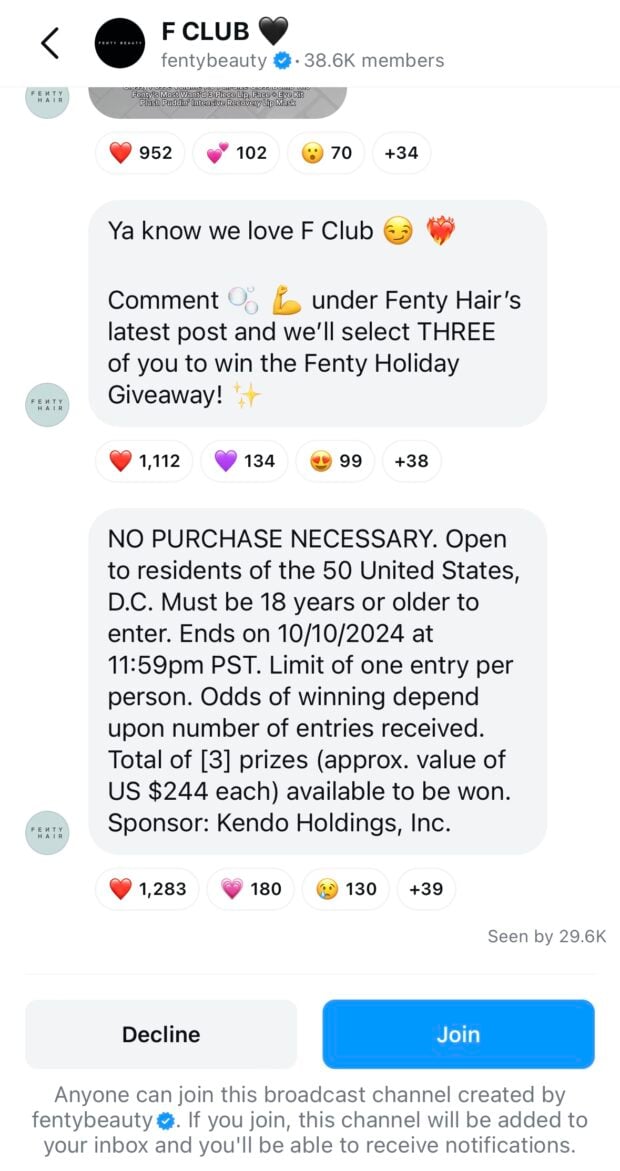
But broadcast channels can also serve as persuasive sales channels. Co-founder and chief brand officer of Moda Operandi, Lauren Santo Domingo, told Vogue Business that the company’s personal shoppers and customer care agents regularly receive preorder requests for products shared exclusively via the brand’s broadcast channel.
To-do list:
- Create shareable content. Work content designed to be shared in DMs (e.g., memes or original insights) into your ongoing strategy. Make sure to include shares in your reporting.
- Consider starting a broadcast channel. If you can offer your most engaged fans something extra (e.g., unpublished content or early access to news), a broadcast channel might be a good place to do so.
- Don’t force it. Avoid unsolicited DMs — instead, encourage organic connections through shareable content that prompts followers to engage on their own terms.
Once upon a time, LinkedIn was known as a stuffy, professional networking site, but my, how the times have changed. In 2025, the platform is redefining itself as the liveliest social platform in town.
From CEOs and industry leaders to creators and recent grads, LinkedIn has evolved beyond networking into more traditional social engagement.
With the addition of new features like polls, a news banner, and even games (yes, you read that right — games!), the platform is working hard to become more appealing to its users. Especially to Gen Z, who will make up 27% of the workforce by 2025.
Brands are taking note of this trend and changing their presence on the platform, too. Businesses are shifting from the formal corporate tone of yesteryear to more relatable, casual, and authentic content that resonates with Gen Z’s preference for transparency and real-world connection.
But it’s not just Gen Z that’s reshaping the platform. Marketers are increasingly seeing LinkedIn as an effective tool for driving website traffic and building brand awareness.
It’s also a great channel for building credibility and meaningful engagement — whether through product launches, Q&As, or behind-the-scenes puppy costume content.
So, it’s not surprising that according to a 2024 survey, LinkedIn is the third most important social media platform for marketers around the globe.
In 2025, more marketers will use LinkedIn to build visibility, attract qualified web traffic, and position their brands as industry leaders.
To-do list:
- Get interactive. Tap into new interactive features like polls, quizzes, and even the new Tango game to boost engagement and grow your following.
- Engage with LinkedIn groups and communities. Stay active in relevant groups to connect with niche audiences and grow your brand’s influence. These communities offer valuable opportunities for brands to engage authentically and establish themselves within specific industry conversations.
- Go beyond professional posts. While LinkedIn was once all business, today’s most successful content is more casual and authentic. Experiment with fun, relatable posts (like your own “puppy parade” video) to humanize your brand and attract a wider audience.
14. UGC creators are the new influencers
The influencer landscape is changing. Gone are the days when brands only looked to big accounts with massive followings for partnerships. Today, businesses are finding more success — and value — working with micro-influencers, nano-influencers, and, most recently, UGC creators.
Unlike traditional influencers, UGC creators don’t need large followings or recognizable personal brands. They’re regular social media users who are paid by brands to create content that looks and feels organic.
These collaborations often include unboxings, tutorials, or lifestyle videos featuring a product naturally, like cooking or beauty routine videos.
There are many reasons to give UGC marketing a shot:
- It offers a cost-effective and authentic alternative to traditional influencer marketing. A piece of UGC content can cost as little as $20! (Plus the advertising budget you use to boost the post.)
- It saves your team’s time, as creators handle content production.
- Most importantly, audiences tend to engage more with content that feels genuine and user-driven, rather than heavily branded.
Win-win-win!
To-do list:
- Experiment with UGC content. Test the impact of UGC-style ads alongside in-house content to see what resonates most with your audience.
- Communicate expectations clearly. Brief UGC creators on any specific details you want them to cover, ensuring alignment with your brand goals.
- Stay transparent. Disclose all paid partnerships. Audiences will appreciate honesty, even in the subtler, organic-feeling ads — and it’s the law.
15. Niche channels are the next big thing
Time to say bye-bye to a one-size-fits-all approach to marketing. The future is all about finding your niche — and creating spaces that feel uniquely tailored to your audience.
It’s clear: exclusive content is on the rise. Brands and creators alike are leaning into private communities and subscription models to meet those evolving expectations.
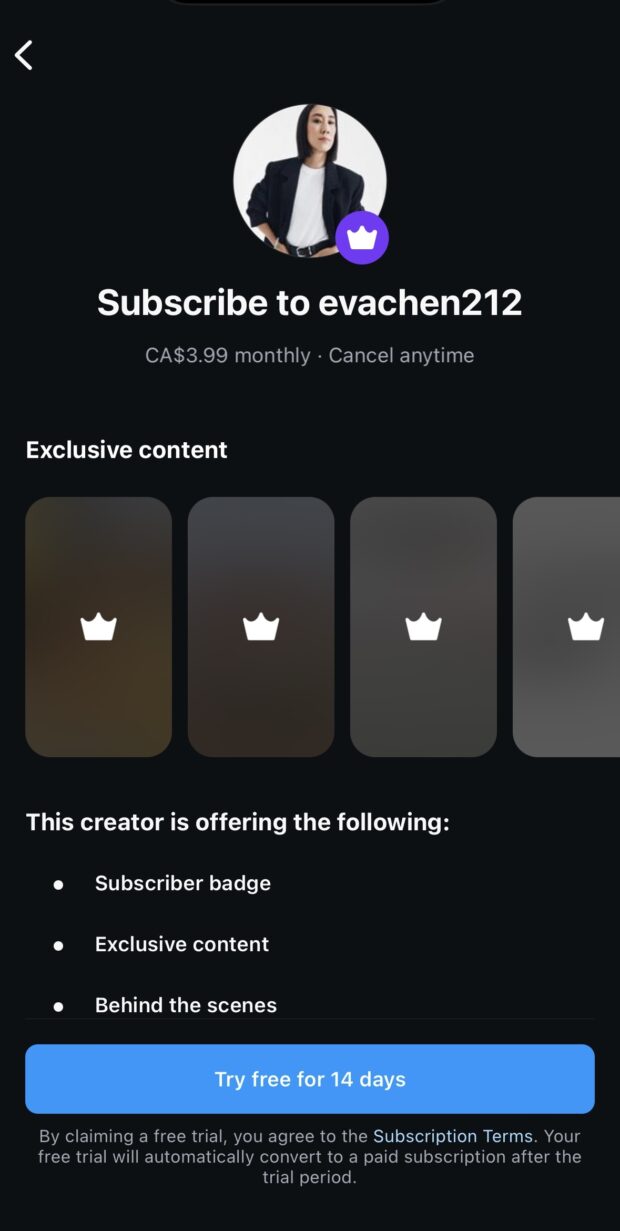
Think Substack, Patreon, Instagram broadcast channels, and even private Facebook groups — all spaces where creators and brands can offer a more intimate, curated experience for their most dedicated followers.
Why put in the effort? Because modern consumers are looking for channels that cater specifically to their interests, where they can connect with brands and creators without the noise of mass-market content.
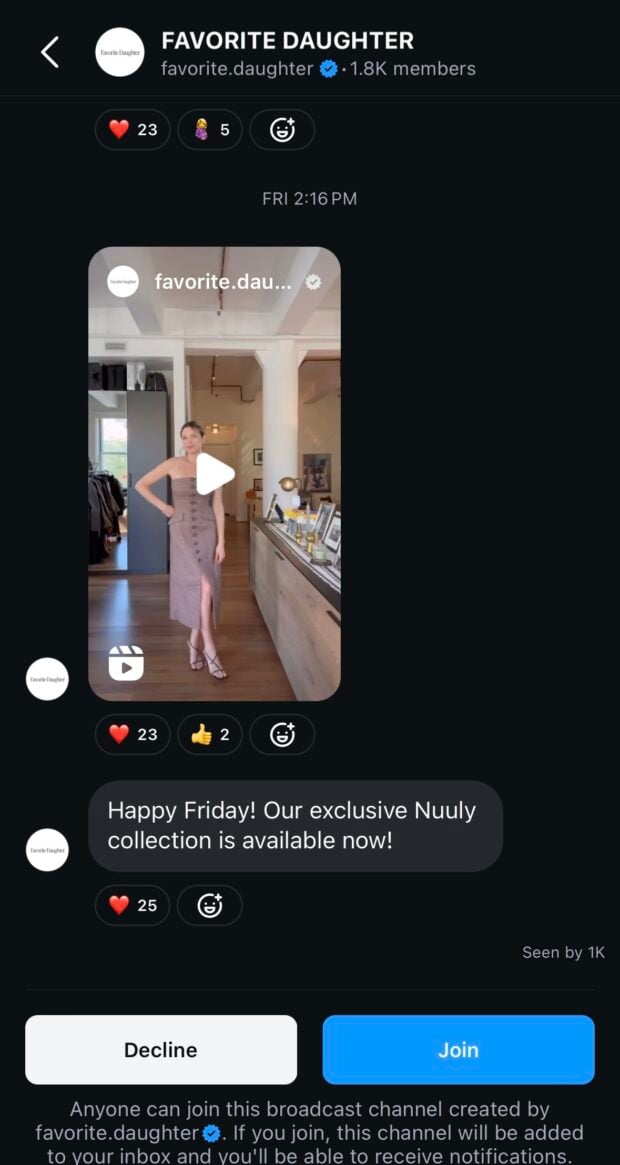
To stay ahead in 2025, invest in more niche channels — whether that’s creating your own private community, offering subscription-based content, or diving deeper into niche platforms that cater to specific interests.
To-do list:
- Test out niche platforms. Explore platforms like Reddit or private Facebook groups where smaller, more engaged communities thrive. Find where your audience already interacts and dive deeper into those spaces.
- Create a subscription channel or private community (like an Instagram broadcast channel) to offer your followers VIP access to exclusive content, such as behind-the-scenes looks or early product releases.
- Personalize your messaging. Craft content that speaks directly to specific segments of your audience using features like Instagram’s “Close Friends” or TikTok’s private posts, making followers feel like they’re getting special attention.
Save time managing your social media presence with Hootsuite. From a single dashboard, you can publish and schedule posts, find relevant conversions, engage the audience, measure results, and more. Try it free today.